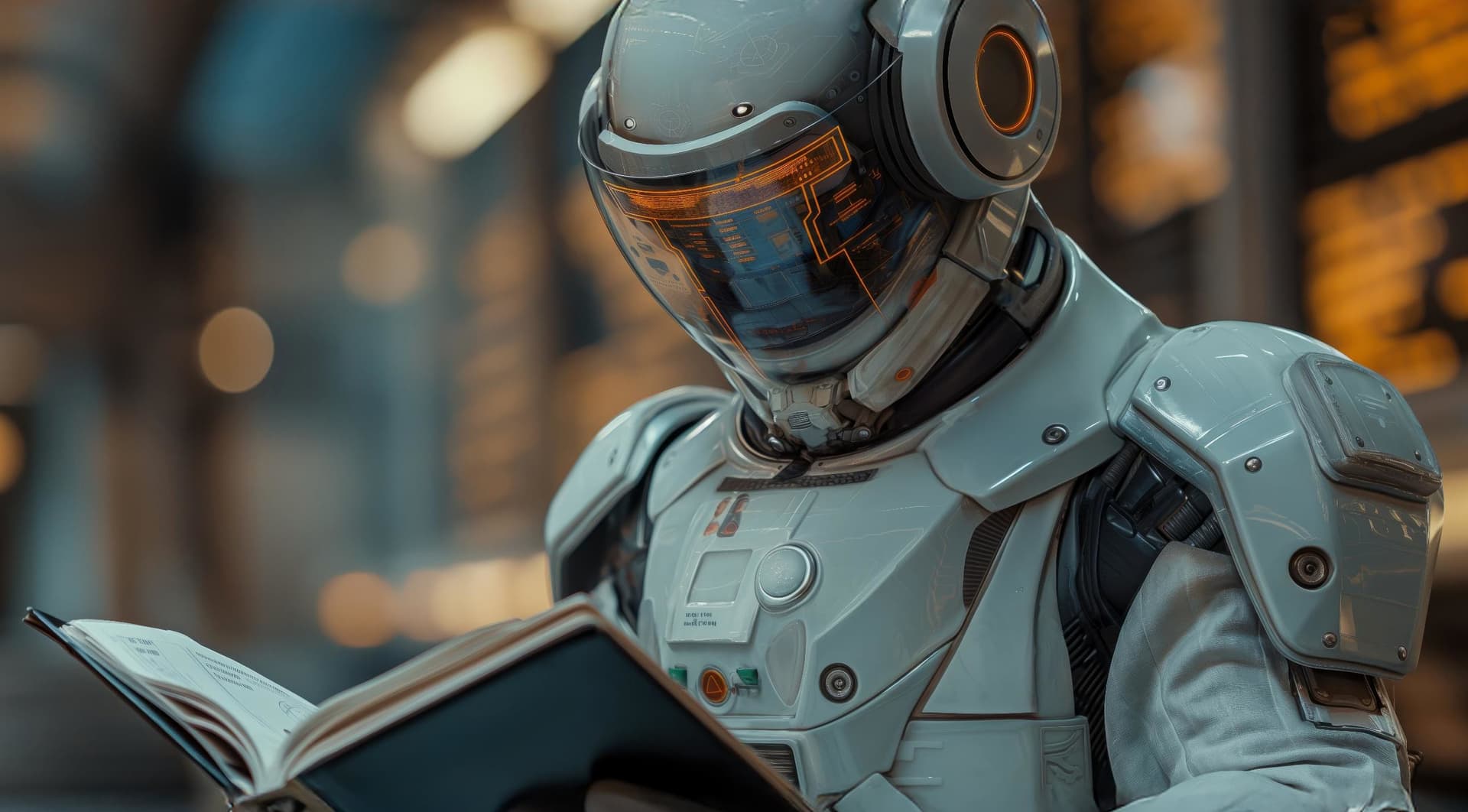
Data labelling
Data labelling is a crucial part of machine learning, where datasets are manually or automatically annotated to train models. These tagged datasets are essential for supervised learning, where the model learns to recognise patterns and make predictions based on labelled examples.
Some examples of how companies are using AI and machine learning for data labelling
Automatic labelling of images in e-commerce
Context: In e-commerce, images of products should be labelled with the right categories, attributes and descriptions to improve findability and provide customers with a better search experience.
Example: A large e-commerce platform uses AI to automatically tag product images with tags such as 'dress', 'blue' and 'cotton'. The AI is trained on a dataset of previously labelled images and learns to automatically categorise and tag new images, saving manual effort and time.
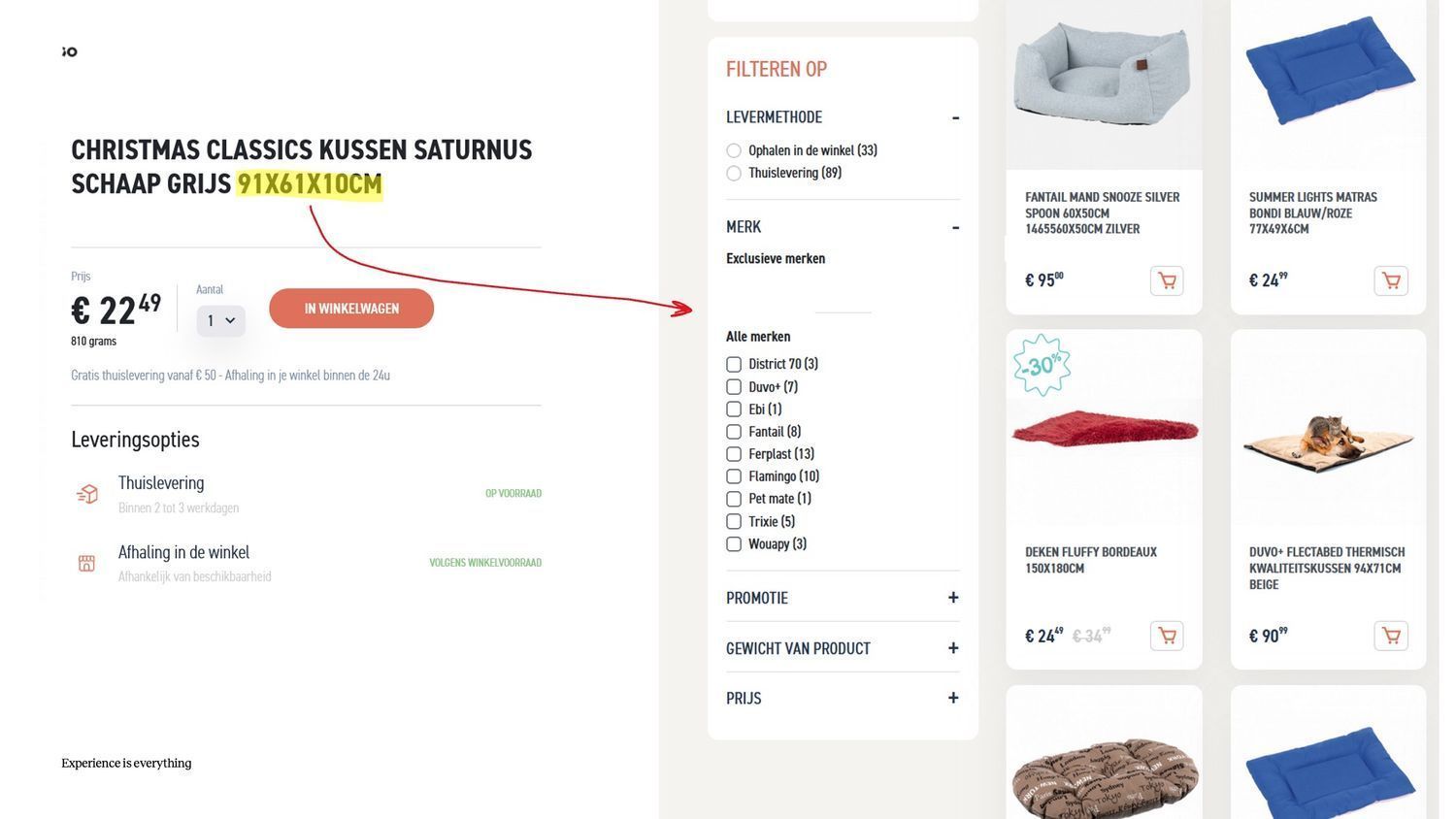
Labelling of voice data for voice recognition
Context: Training speech recognition systems requires labelling large amounts of speech data with appropriate transcripts and intentions.
Example: A company developing digital assistants uses machine learning to automatically transcribe and label voice recordings with the appropriate text and voice commands. This labelled data is then used to improve the accuracy of their voice recognition models.
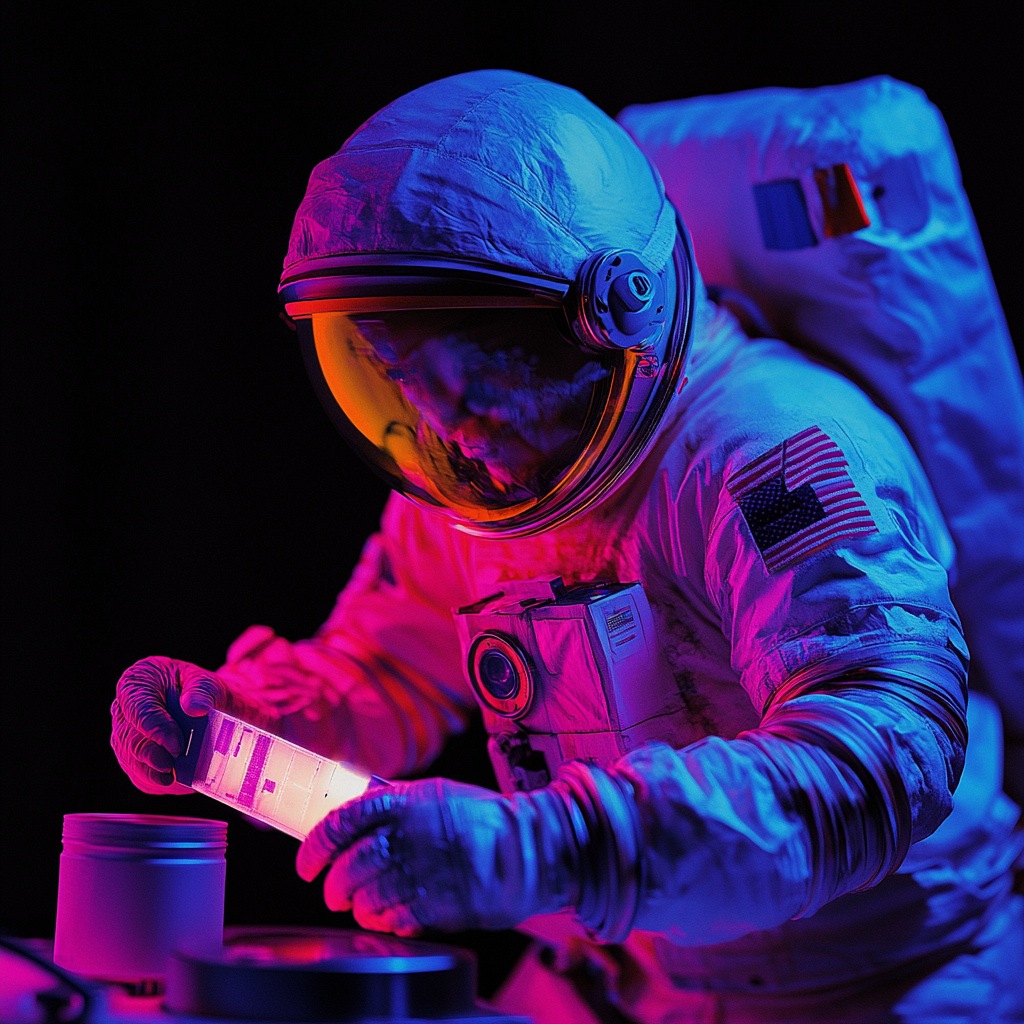
Labelling videos for autonomous driving
Context: In the development of autonomous driving vehicles, it is essential to tag video data with objects such as pedestrians, vehicles, traffic lights and road markings.
Example: A company developing self-driving cars uses AI tools to automatically annotate video recordings of traffic situations. The system recognises objects such as cars and pedestrians and labels them in the video footage, helping the AI models better learn how to react in different traffic situations.
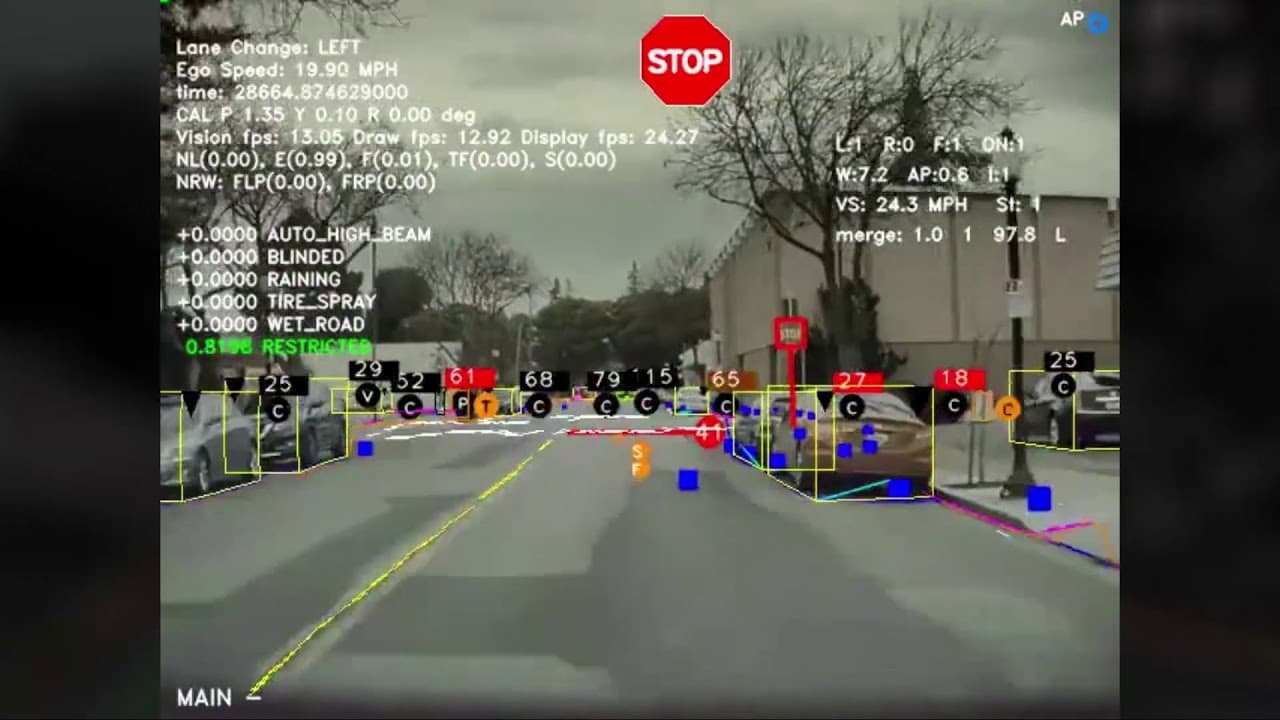
Labelling of medical imaging for diagnostics
Context: In healthcare, medical images, such as MRIs and X-rays, are labelled with the presence of specific conditions or abnormalities to train AI models that support doctors in diagnostics.
Example: A hospital uses machine learning to automatically label MRI scans with the presence of tumours. The AI is trained on thousands of labelled scans and helps radiologists identify abnormalities quickly and accurately.
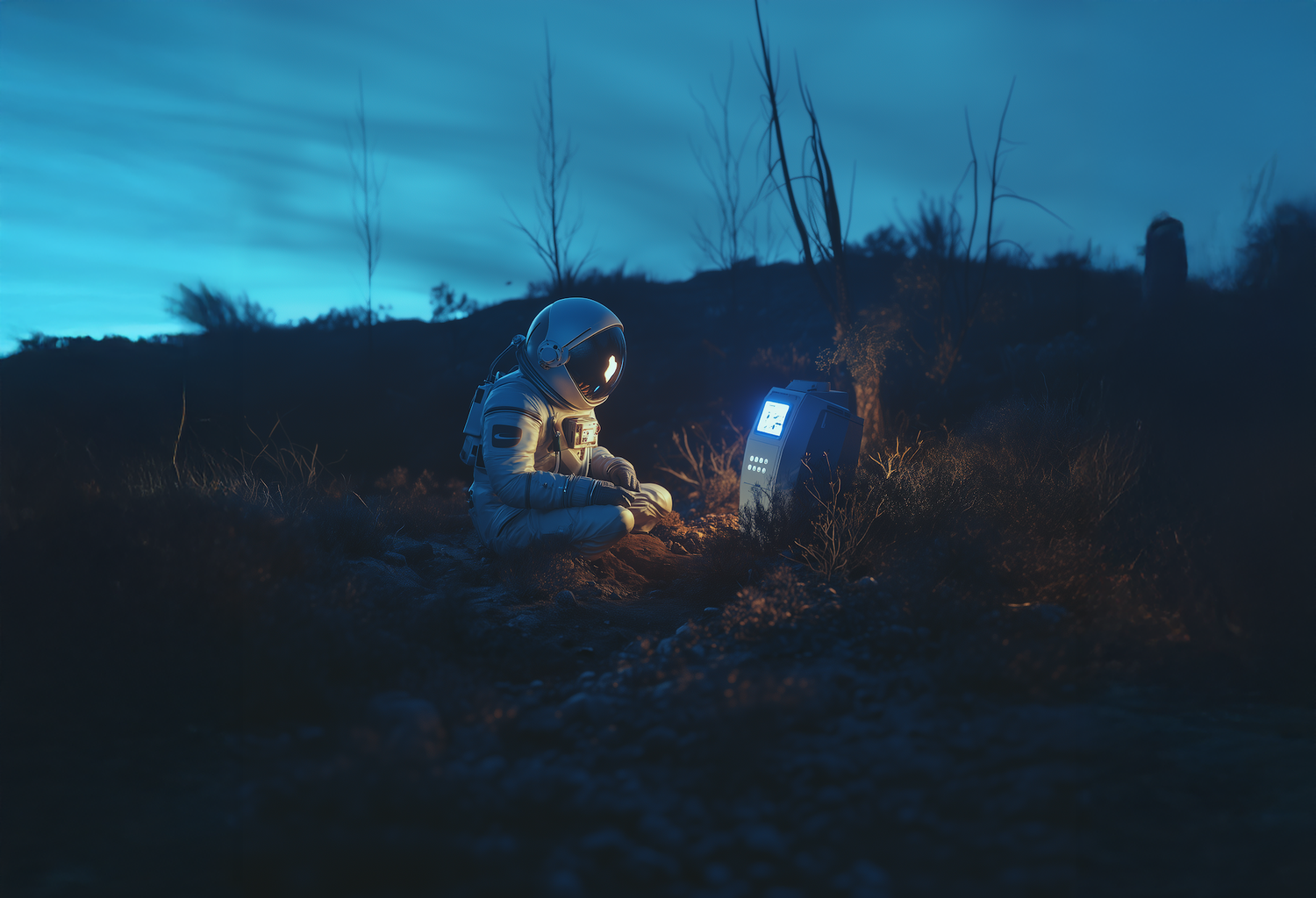
Labelling of fraudulent transactions for fintech
Context: To detect fraudulent activity in financial transactions, companies need to label datasets with examples of fraud and non-fraud.
Example: A fintech company uses AI to label large amounts of transaction data as 'fraudulent' or 'non-fraudulent'. The AI learns from this labelled data to identify suspicious transactions and prevent them from happening.
Labelling of textures in the manufacturing industry
Context: In the manufacturing industry, images of products can be labelled with appropriate textures and patterns to automate quality checks.
Example: A textile manufacturer uses AI to automatically label pictures of fabrics with texture characteristics such as 'smooth', 'ribbed', or 'knitted'. These labels help control product quality and consistency in the production process.
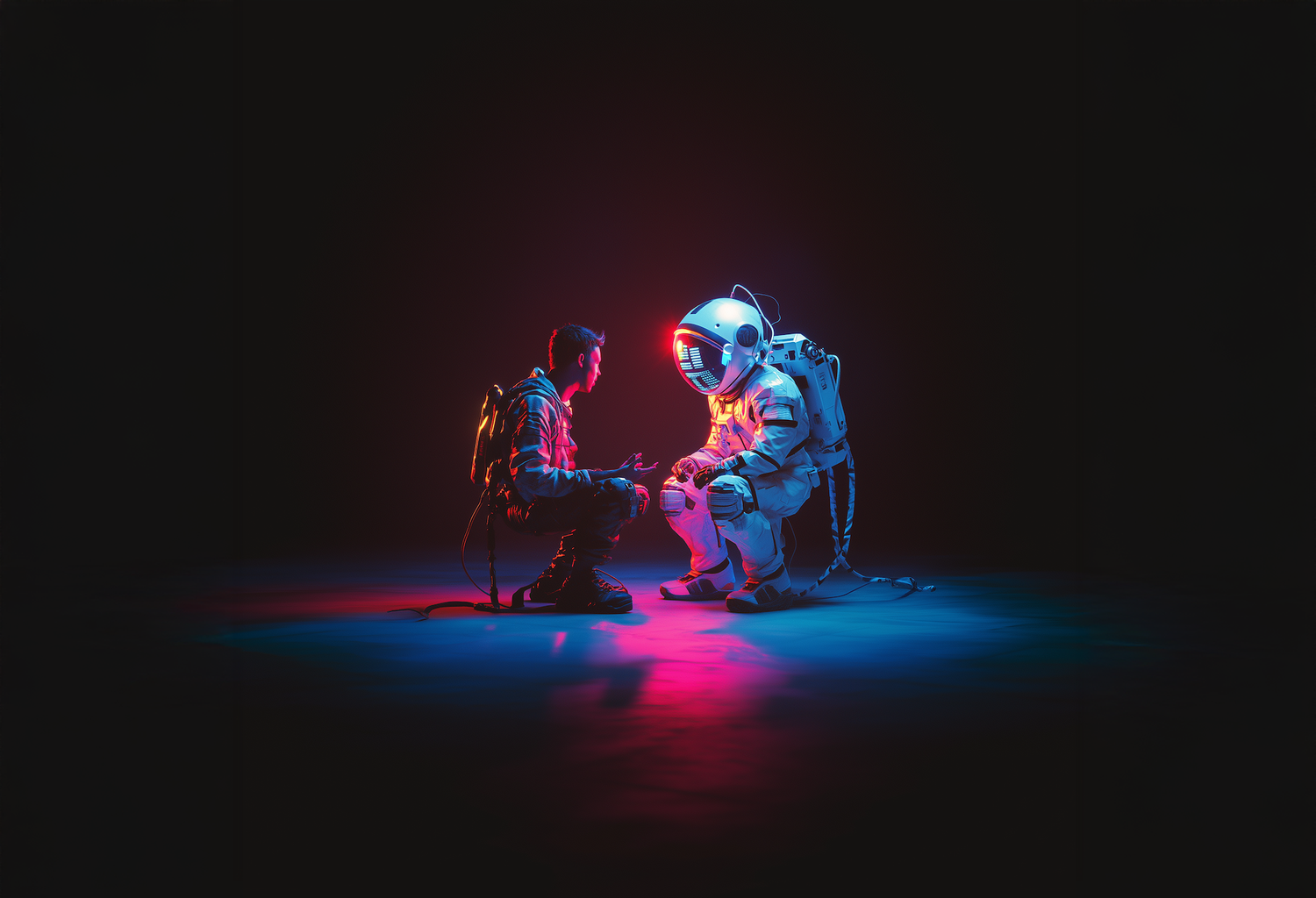
Labelling natural language for chatbots
Context: To develop effective chatbots, companies need to label datasets with intentions and entities that correspond to specific user queries and commands.
Example: A customer service company uses AI to analyse customer call logs and automatically label them with the correct intentions, such as 'place order' or 'request account information'. These labelled datasets help train chatbots to better understand and correctly answer customer queries.
Labelling of geolocations in mapping services
Context: Maps and navigation services require geolocation data to be labelled with descriptions such as street names, places of interest and directions.
Example: A navigation app uses AI to automatically tag satellite images with geolocations such as buildings and roads, which helps improve map accuracy and route planning.
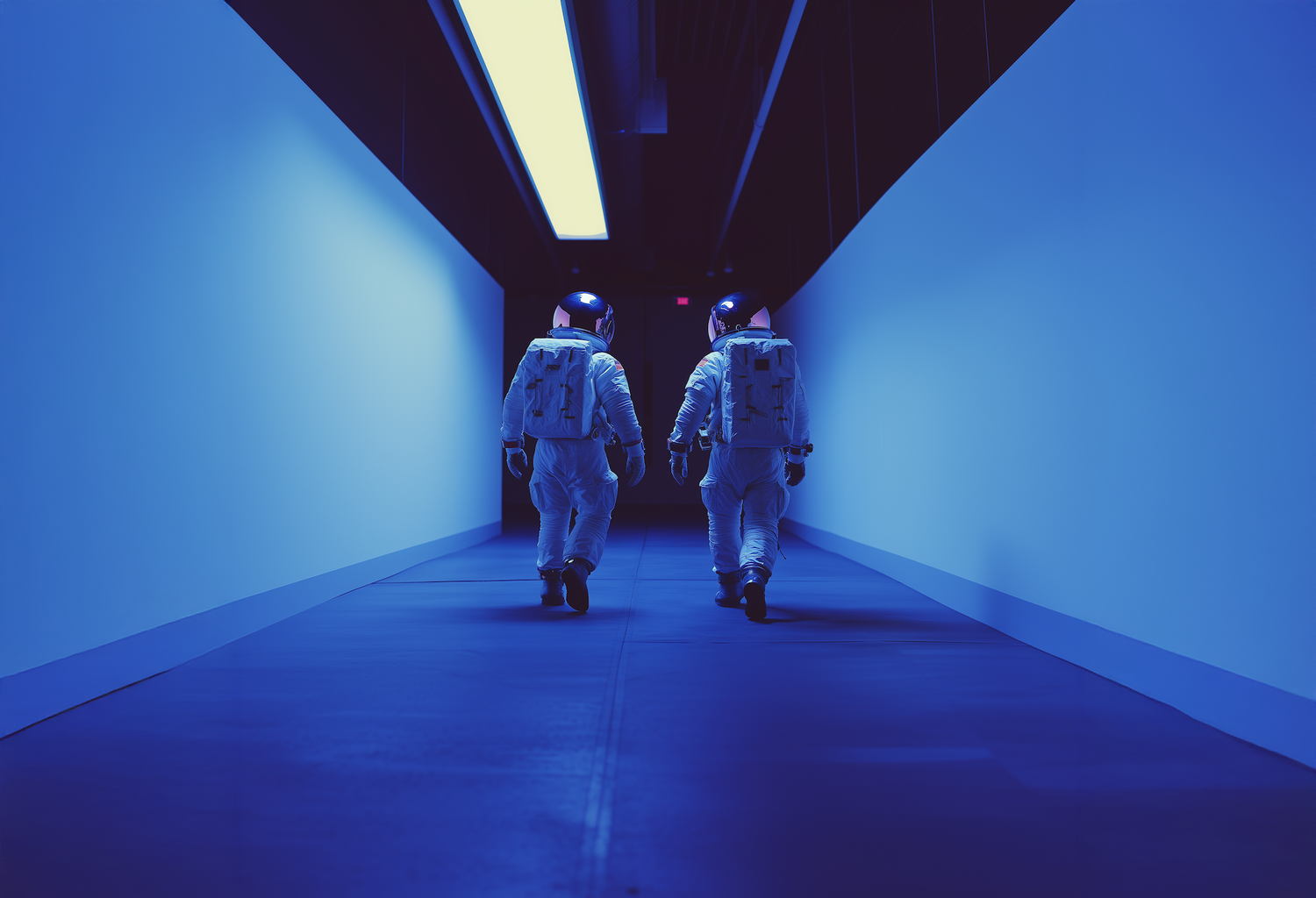
Labelling of content in content monitoring
Context: On social media and other online platforms, content should be labelled to identify and remove harmful or inappropriate content.
Example: Social media use AI to automatically label posts, images and videos as 'appropriate' or 'inappropriate'. These labelled datasets are used to train the AI models that monitor content and protect users from harmful content.