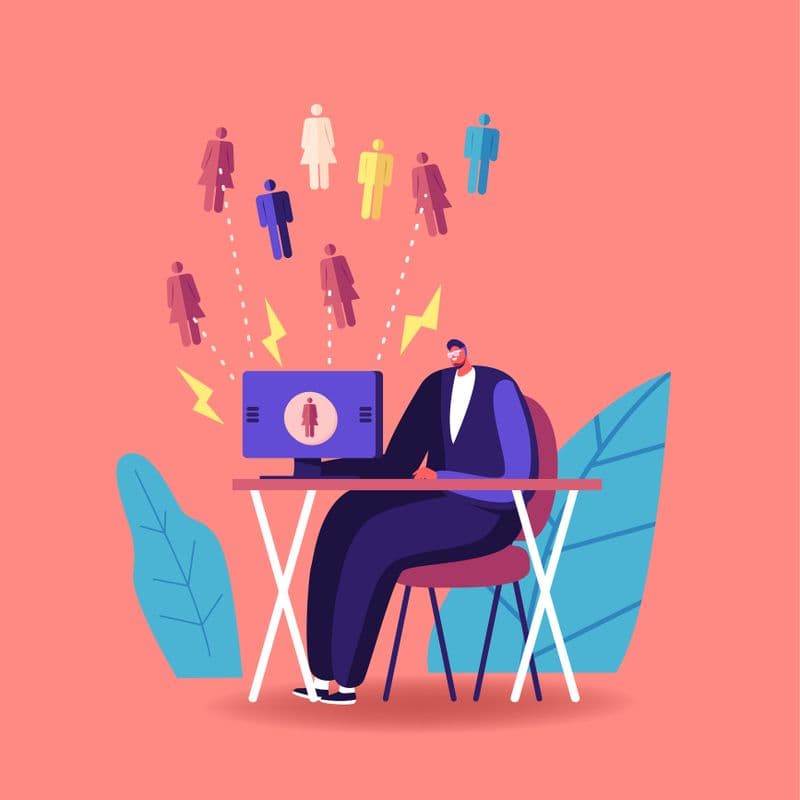
Blog
Historical data export - Universal Analytics
Deadline for exporting Universal Analytics: 1st of July 2024
After the 1st of July 2024, it will no longer be possible to access data in Universal Analytics. Therefore, it’s essential to take timely action and export the data before that day.
By exporting the data before the access is closed, you can gain valuable insights, store the data securely and take any further actions. It is wise not to do this at the last minute, as there may be obstacles, such as errors in extraction or technical challenges.
To avoid potential problems, it is advisable to transfer the data in time to environment where it can be stored securely. That way, you can later use the data for further analysis or transformation purposes. By acting now, you can ensure that you don’t lose the valuable data and are well prepared for the future.
So don't wait until the last minute - make sure you export the data from Universal Analytics before 1 July to a secure environment, where you can continue to use it.
Use cases for historical data retention
Retaining historical data offers several valuable use cases that can help you make strategic decisions and optimise your business performance. Here are some key use cases where retaining historical data can be of great value:
Trend analysis over years: By analysing historical data, you can identify trends and gain insights into the evolution of your visitors' behaviour. For example, by looking at the frequency of visits over the years, you can discover patterns and understand how your users' engagement evolves. This allows you to develop and optimise customer retention and engagement strategies.
Impact of GDPR: With the introduction of GDPR, there may have been changes in the data available and how it is captured. By keeping historical data, you can analyse which part of the data is still recognisable and which data has been lost due to the new regulations. This insight will help you assess the relevance and accuracy of your analytics and make any adjustments to comply with GDPR.
Changes in visitor demographics: Historical data can provide valuable insights into how the demographics of your visitors have changed over time. By understanding these changes, you can better tailor your marketing strategies to the needs and interests of your target audience. You can identify new segments and develop personalised campaigns to increase engagement and conversion.
Repeated campaigns: If you have invested heavily in past campaigns that are repeated annually, retaining historical data is essential. By analysing the performance of these campaigns in previous years, you can gain insights into what worked and what didn't. This will help you further optimise your marketing strategies, increase ROI, and avoid any past mistakes.
Mapping seasonal effects: Seasonal effects can affect the behaviour of your visitors and the performance of your website. By analysing historical data, you can map these seasonal effects and understand how they affect your business. This allows you to align your marketing and sales efforts with these seasonal fluctuations and plan your resources effectively.
Retaining historical data thus offers several advantages. By using historical data, you can make better-informed decisions with the end goal of achieving set goals for your organisation.
What to look out for and consider?
When comparing Google Analytics 4 (GA4) and Universal Analytics (UA), there are some important aspects you need to consider making the right choice for your analytics needs.
Here are some things to keep in mind:
Different data model: The main difference between GA4 and UA lies in the way they collect and report data. GA4 is event-based, meaning it focuses on capturing specific events and interactions on your website. UA, on the other hand, uses composite metrics and is more focused on tracking sessions and page views.
Session and user numbers: It is important to note that session and user numbers may differ between GA4 and UA due to the different methods of data collection. UA uses cookies to track sessions and users, while GA4 takes a more flexible and privacy-friendly approach using events and anonymised user IDs. This can lead to differences in reported numbers, so it is important to take this into account when comparing data.
Impact of anomalous events such as COVID-19: Anomalous events, such as the COVID-19 pandemic, can have a significant impact on historical trends and data. It is important to understand that historical trends may not be representative of normal behaviour during this exceptional period.
Understanding the difference between GA4 and UA, evaluating session and user numbers, and considering anomalous events such as COVID-19, are important factors when making a decision.
By storing data in BigQuery, you can apply data transformations, making the data model of both analytics platforms comparable. With this, you enable trend analysis and other analytics to be drawn up more fairly between the platforms.
Why you can't just save everything from Universal Analytics
While UA is a powerful tool for collecting and analysing data, there are limitations to consider when storing all available data. Here are some reasons why you can't just save everything from Universal Analytics:
Reporting API limitations: Universal Analytics' Reporting API has limitations in terms of report size. Reports can consist of a maximum of 10 dimensions and 9 metrics. This means that not all available dimensions and metrics can be included in a single report. When creating the data schema for exporting UA data, it is important to determine which dimensions and metrics are most relevant to your specific needs and which reports you want to generate.
Focus on relevant reports: Storing all available data can lead to an abundance of information that may not all be relevant to your analytics and reporting needs. It is important to determine which reports are most valuable and useful for your business. By defining specific reports and storing only the necessary dimensions and metrics, you can focus on the data that really matters and work more efficiently.
At iO, we have meanwhile worked out a data scheme that takes these shortcomings into account. Based on this, you obtain interesting UA data with which analyses are possible. The scheme below shows the output in the form of datasets:
Which way to store Universal Analytics
Moving Universal Analytics data to BigQuery is a valuable step to better manage and leverage your data. Using Airbyte OS, installed on a virtual machine in Google Cloud, we are going to simplify and automate this process. Through this ETL Tool, the data from UA – based on the data scheme we set out – will be loaded into BigQuery. This will enable you to manage, analyse and leverage your UA data for marketing insights and decision-making more effectively.
Contact
Feel free to contact us for support in setting up and configuring Airbyte OS and moving your UA data. We are ready to help you through every step of the process.
Future-ready with your own Marketing Data Hub
Setting up an ETL tool with Airbyte on a virtual machine in Google Cloud and using a marketing data hub can be a powerful approach to store and leverage data for marketing purposes. Here is an overview of the vision behind using a marketing data hub:
Marketing cloud environment: The marketing data hub acts as a consolidated environment where all marketing data can be collected and edited. It provides a central hub where all your marketing data comes together, giving you a holistic view of your marketing activities.
Single source of truth: By using a marketing data hub, you create a single source of truth for all your marketing data. As a result, you avoid inconsistencies and duplicate data, and you can rely on the accuracy and consistency of your data.
Flexible and scalable: A marketing data hub allows you to pay only for what you use, allowing you to optimise costs. Moreover, integrating data from other platforms allows you to get a more complete picture of your marketing efforts and make better decisions.
Own server for server-side tagging: With a marketing data hub, you can set up your own server for server-side tagging. This allows you to manage the tracking and collection of data and adapt it to your specific needs.
Ability to install data tools: By using virtual machines in the cloud, you can easily install and use data tools on your own cloud server. This gives you full control over your data infrastructure and allows you to integrate specific tools and technologies relevant to your marketing goals.
Data transformation with Dataform: Using Dataform, you can transform data and prepare it for analysis and reporting. This allows you to perform complex transformations and structure your data in a way that is most valuable for your marketing activities.
Futureproofing with cloud tools like Vertex AI: By using cloud tools like Vertex AI – which enable machine learning and AI – you can prepare for the future of marketing. For example, these tools can generate smart product recommendations based on advanced algorithms and patterns in your marketing data.
Ready for the future with data?
If you are interested in implementing an ETL tooling with Airbyte and setting up a marketing data hub, we encourage you to contact our team. We can help you set up the right infrastructure and implement the necessary tools to effectively manage and leverage your marketing data for better marketing insights and decision-making.